
Unlike the use of external data in conventional analysis, the value of AI and machine learning differs greatly when external data changes its shape and persists as a learning result.
This consortium will tackle various issues surrounding AI, including annotations.
Intellectual Property / Contract
Study intellectual property and contracts of training data used in AI (deep learning) / ML (machine learning), and create contract model templates with "Smart Contract" mechanism.
AI Research
This working group will examine data that contributes to AI research and how to solve social issues using collected data with latest AI technologies.
Data Collection and Utilization
Activities that contribute to the promotion of data trading, sharing and utilization by data holders, annotators and data consumers such as researchers and data scientists.
Data Platform
Build data platform which provides functionalities needed for trading, smart contract, controlled data access, policy enforcement and provisioning. Also the platform will provide computation resource for AI/ML.
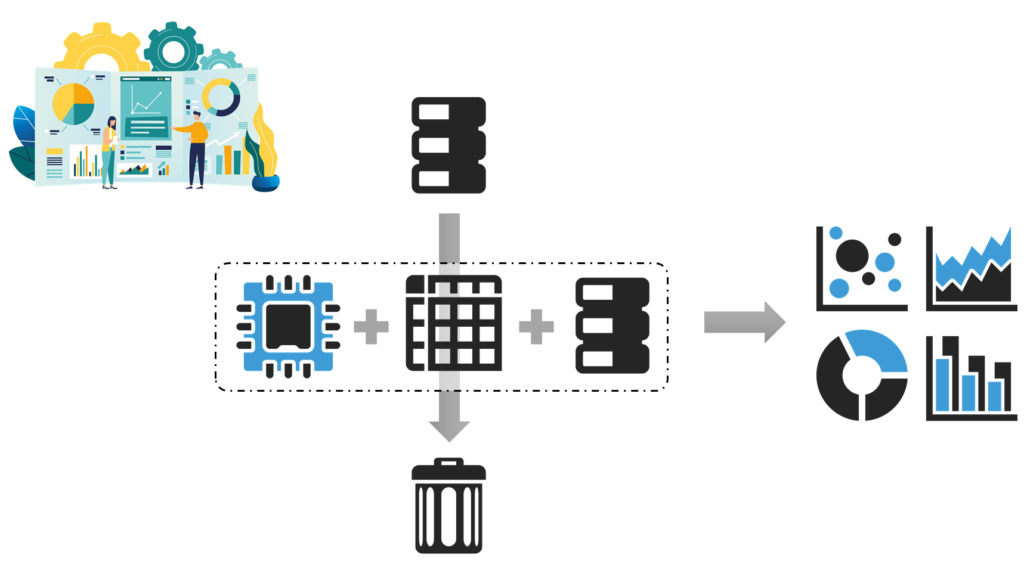
Data transaction on the premise of conventional disposable
Most of the external data use by general data analysis such as sales analysis is one-time use and finishes its role as work is completed. As such, current data trading model and contracts assume that the data does not directly create secondary value. (External data finishes its role with the completion of analysis, and the original data does not appear in the analysis results in principle. Data consumers are facing risks by using data for AI/ML under traditional data trading practices.)
AI / ML where data transforms and persists
Training data set transforms and persists in AI/ML model, and have secondary value which is different from its original data set.
As traditional data trading model and contract does not assume the use of data for AI/ML whose usage and technology are very different compare to traditional usage. Thus data holders and consumers are holding risks that are not well known.
When using external data for AI/ML training, the provenance of data including accountability (product liability) must be considered in addition to intellectual property (patents, copyrights, design rights, trademark rights).

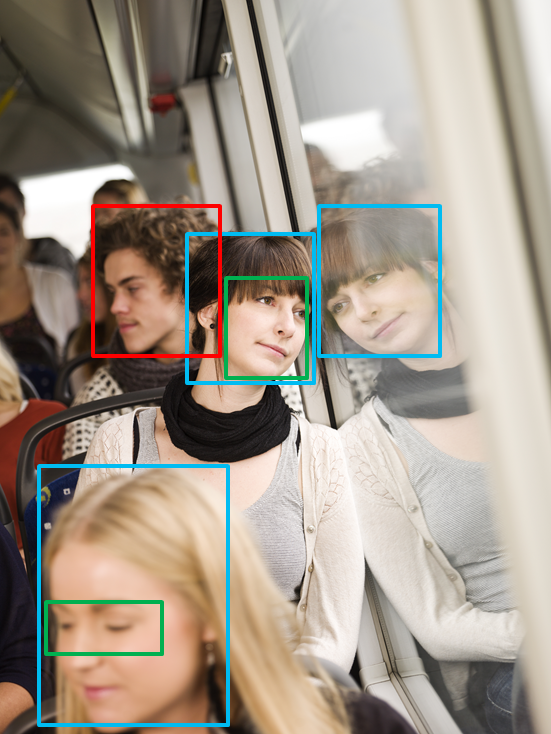
Different annotation criteria depending on the problem
Different annotation specifications vary depending on the problem. When not only original data but also cleansed and annotated data are to be distributed, it is important to clarify the specifications, definitions, and standardization.
The AI Data Consortium will also examine data cleansing, annotation specifications, standard formats, etc., in order to promote smooth sharing and distribution of data.
Transparency and Provenance are Critical
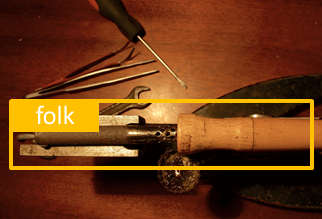
Label soldering iron as folk
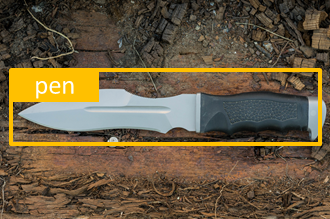
Label knife as pen
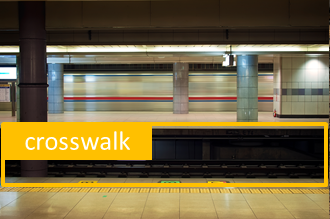
Label railroad as crosswalk
The importance of ensuring the accuracy of data and the location of responsibility for the consequences are increasing as AI is built into the system. How to prevent malicious cleansing, annotation, and unintended data bias. It is becoming increasingly important as one of the security surrounding AI.
Data Provenance
- When, who, where and how the data was created
- When, who, where and how the data was cleaned
- When, who, where and how the data was annotated
- When, who, where and how the data was evalutated

Data history management is getting important